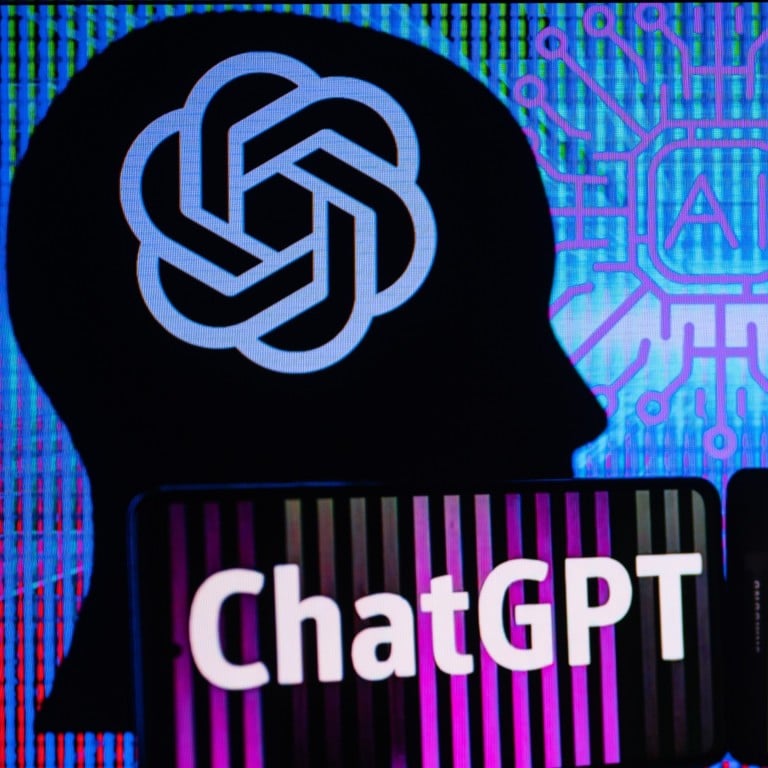
Chinese scientists’ attack on ChatGPT shows how criminals can use weak spots to exploit AI
- Using researchers’ attack method, a giant panda’s face is misclassified as a woman by Bard and a bald eagle is misclassified as a cat and a dog in Bing Chat
- Researchers hone in on models’ vulnerabilities as world’s powers sign up to Bletchley Declaration at UK summit on AI safety
Doctored images used by the researchers appeared almost identical to the original, but they could effectively circumvent the models’ mechanisms designed to filter out toxic information.
The findings highlight significant security concerns within artificial intelligence and help shed light on the vulnerabilities of commercial multimodal large language models (MLLMs) from tech giants including Google, Microsoft and Baidu.
Wu Zhaohui, China’s vice-minister of science and technology, took part in the conference and presented proposals, advocating for stronger technical risk controls in AI governance.
Zhu Jun and Su Hang from Tsinghua University’s department of computer science and technology said in a paper that criminals could exploit these inherent AI vulnerabilities to produce harmful content. The paper did not detail how those weaknesses could be exploited.
“As large-scale foundation models such as ChatGPT and Bard are increasingly utilised for various tasks, their security issues become a pressing concern for the public,” they stated in their paper published on the arXiv website in October.
Baidu launches paid version of Ernie Bot as firms look to cash in on AI chatbots
The research team outlined two types of adversarial attacks against MLLMs: image feature attack and text description attack.
The former changes the features of a sample, affecting subsequent judgments. The latter attacks the entire process, resulting in generated descriptions that differ from the correct ones, thereby confusing the machine.
The adversarial attacks made minute, almost imperceptible, changes to the original picture. To the human eye, the altered samples showed almost no difference to the original image.
Bard – with its face detection and toxicity detection mechanisms – actively rejects images containing faces or violent, bloody, or pornographic content to protect privacy and prevent abuse.
The overall decision-making process of MLLMs is a “black box”, with its architecture and parameters remaining unknown. However, the algorithms for face and toxicity detection are familiar to scientists with researchers launching attacks on these sub-models.
The team applied similar mathematical manipulations and manually collected photos to attack Bard. According to their paper, 38 per cent of images bypassed the face detector, and 36 per cent evaded the toxicity detector.
For instance, in a breach of its own privacy protections, Bard identified a Korean singer and provided detailed content descriptions for an image of a group of soldiers holding guns, which contained violent information.
The experiment emphasises the potential for malicious attackers to use Bard to generate inappropriate descriptions of harmful content.
China’s AI talent in short supply amid surging demand triggered by ChatGPT race
These findings suggest that most MLLMs have vulnerabilities in their image content recognition.
From the code the Chinese team provided with the paper, 200 generated adversarial examples can mislead AI models to output the wrong image descriptions, with a 22 per cent success rate against Bard, 26 per cent success against Bing Chat and an 86 per cent attack success rate against Ernie Bot.
“The current defence mechanisms of Bard can be easily bypassed by adversarial examples, highlighting the need for targeted defences to ensure the safety of MLLMs,” the team said in the paper.
However, there is an imbalance between research into attacking and defending AI models. An anonymous researcher said most annual publications on adversarial models focused on attacks, with only a few investigating defence.
“This is because a single defence strategy may only be effective against one type of attack, and it is far more difficult to defend against all potential attacks than to target a fixed objective,” he said.
“Traditional defence methods that increase robustness might lead to a trade-off in accuracy and can be computationally expensive, making them challenging to apply to large models,” Zhu stated in the paper.
Pentagon unveils AI strategy to boost US ‘decision advantage’ against China
He suggested that preprocessing-based defences might be more suitable for large-scale foundation models.
Despite extensive research, defending against adversarial attacks on vision models remains an unresolved issue.
“We hope this work can deepen our understanding on the robustness of MLLMs and facilitate future research on defences.”
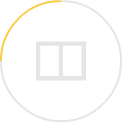